Unlocking Cancer’s Secrets Using the ‘Social Networks’ of Cells
Megha Padi, PhD, of the UArizona Cancer Center has developed a method for probing the genetic underpinnings of cancer and other diseases, which could lead to better treatments.
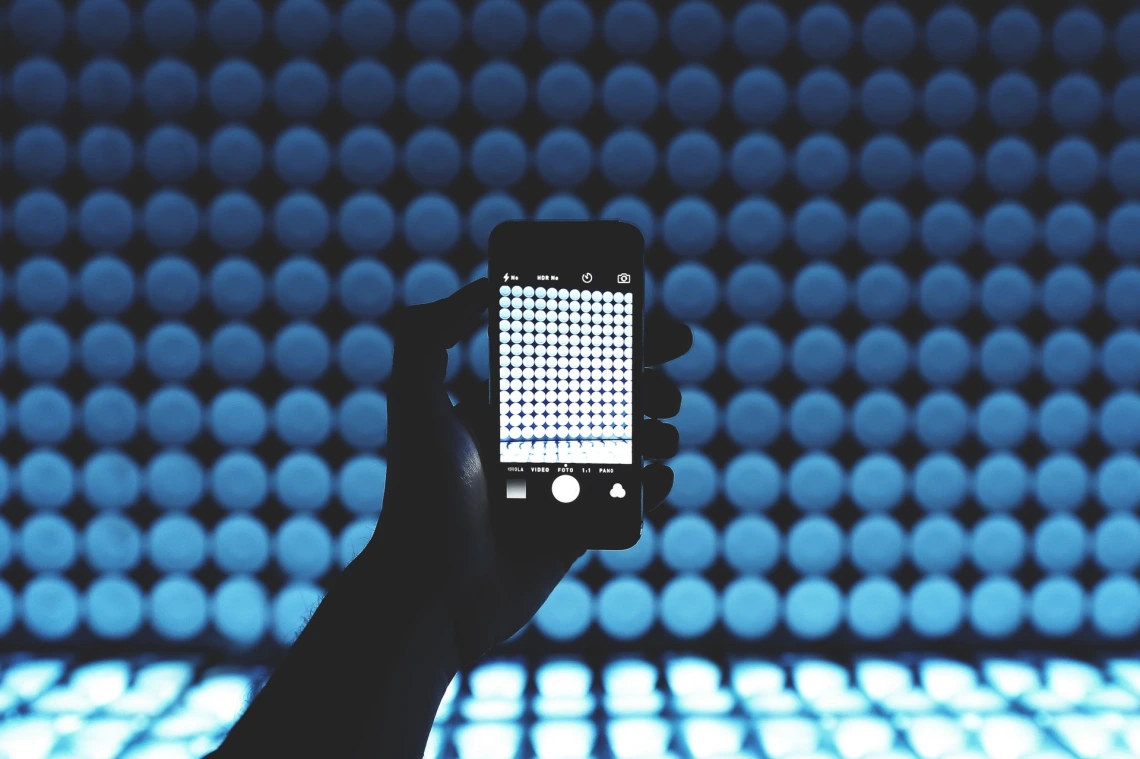
Recent headlines have cast suspicion on social network analysis, which can mine data from the internet to target advertisements or potentially influence elections.
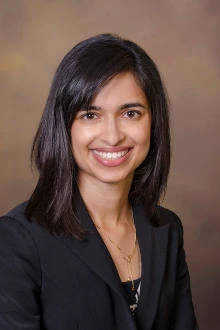
Megha Padi, PhD
But what if we could use those same tools not for the economic or political gain of a few, but for the health of all humankind? Scientists now can harness the tools of social network analysis to understand connections among genes, an advance that someday could enable medical discoveries.
Megha Padi, PhD, director of the University of Arizona Cancer Center Bioinformatics Shared Resource and an assistant professor of molecular and cellular biology, developed a computer algorithm called ALPACA that reveals which gene networks are activated in a diseased cell — an approach that could lead to better treatments for various diseases. The results were published in the open-access Nature Partner journal Systems Biology and Applications.
Cancer researchers usually focus on specific genes when comparing healthy cells to tumor cells, an approach that does not completely explain what occurs behind the scenes to cause cancer.
“You can get a list of the parts in your car, but you won’t understand what makes the car run until you understand how all the parts are connected to each other,” Dr. Padi says.
Likewise, it is essential to study how genes work together as part of a larger network, so Dr. Padi is analyzing these gene communities in the same way one would examine a social network composed of connections among people who know one another.
Dr. Padi is the first author on the study, in collaboration with John Quackenbush, PhD, then-director of the Center for Cancer Computational Biology at Dana-Farber Cancer Institute. The study was conducted when she was a postdoctoral fellow at Dana-Farber; Dr. Padi joined the University of Arizona in January 2018.
Gene ‘communities’
Genes in a community, like people in a social network, talk to one another. In a healthy cell, gene communities function like factory workers, cooperating to process raw materials into goods the cell needs to thrive. In a diseased cell, miscommunication along the “assembly line” results in defective products.
Tracking how genes’ conversations change over time might provide clues about how cancer arises. These conversations can be analyzed using tools developed to study social networks.
“A classic example is a phone network,” says Dr. Padi. “I’m calling my mom, my mom may be calling my sister, my sister’s calling me, and I may call you, but you won’t call my mom. Natural communities are formed, like your work community and family community.”
Dr. Quackenbush adds, “In the same way, we see that gene regulatory networks form communities. The pattern of ‘conversations’ within the communities change between healthy and diseased individuals. ALPACA is the first method to understand how the cell’s ‘social network’ is reorganized in disease, which might provide clues how cancer forms.”
“You can get a list of the parts in your car, but you won’t understand what makes the car run until you understand how all the parts are connected to each other.”
Megha Padi, PhD
To uncover cancer’s causes, the challenge is to find the differences between gene communities in healthy cells compared to diseased cells, rather than the differences between individual genes. But comparing gene communities is easier said than done.
Disentangling the ‘hairball’ with network analysis
The genetics underlying cancer can be complex, with tens of thousands of interacting components to sort through. Drawing a diagram of these interactions results in what researchers call a “hairball.”
“To make a map that human beings can actually understand, scientists need computers to figure out the subtle ways in which this ‘hairball’ goes awry in tumor cells,” Dr. Padi says.
ALPACA’s incorporation of social network analysis is an innovative use of tools that are most commonly associated with marketing, not medical research.
“Network scientists usually ask questions like how information is being spread through Twitter or other communication channels,” Dr. Padi says. “We’re asking completely different questions, like how networks function in different types of tumors. This type of research is rare because not many people work on both those fields at the same time. Network medicine is still an up-and-coming field.”
Dr. Padi’s approach enables a more holistic exploration of the genetic underpinnings of cancer.
“The method allows us to sift through the ‘hairball’ and pinpoint the regulatory pathways that drive disease,” says Guang Yao, PhD, UArizona assistant professor of molecular and cellular biology, who was not involved in developing ALPACA. “There are many statistical methods for finding changes in individual genes, but we ultimately want to understand the mechanism that drives these changes.”
Scientists now can harness the tools of social network analysis to understand connections among genes.
Finding better treatments
Understanding what causes cancer is an important scientific question in its own right, and can benefit patients if this knowledge is harnessed to find better treatments. One of the next steps is to identify drug candidates that can be further investigated in the laboratory.
“We’d like to use what we have learned to develop new strategies that can help prevent or cure disease,” says Dr. Quackenbush.
Dr. Padi is particularly interested in using ALPACA to find novel treatments for people whose cancers fail to respond to currently available treatments. By contrasting cancers that cannot be treated with drugs, known as chemoresistant tumors, with chemosensitive tumors, those that can be treated with drugs, researchers may be able to zero in on a community of “bad guys” — genetic pathways that might be targeted with customized drugs.
‘Big data’ births a new generation of case-control studies
Case-control studies compare a group of people with a disease (cases) to a group of similar people without the disease (controls). This study design gained prominence in the 1950s when it was used to demonstrate the strong association between smoking and lung cancer.
Dr. Padi is offering ALPACA as a tool for analyzing genetic sequencing data from case-control studies, allowing researchers to identify gene communities that are active in disease but not in healthy cells. For example, after analyzing data from ovarian cancer cells, ALPACA was able to pinpoint known drug targets in ovarian cancer, showing that it is returning valuable information, rather than random noise, that could help treat disease.
“We’ve shown that ALPACA does reveal mechanisms you can target with drugs that are known to be effective.” explains Dr. Padi. “Now you can run this on other data sets where we don’t know what we expect to find, and potentially find something new.”
Using “big data” and network analysis to analyze case-control studies is a modern twist on a classic study design.
“Before this, there’s never been a method that could compare genome-scale networks in a case-control manner,” Dr. Padi says. “There’s a lot of work to do in this area.”
Please Note: Drs. Padi and Quackenbush used databases developed with information from patients who consented to have their anonymized data used for research. This research was supported by NIH grants K25 HG006031, R01 HL111759 and R35 CA197449.
Our Experts
Contact
Health Sciences
Office of Communications
520-626-7301
public@arizona.edu