A Simple Blood Test to Speed Pulmonary Hypertension Diagnosis
Health Sciences researchers are using artificial intelligence to quickly identify the chemical fingerprints of pulmonary hypertension before it’s too late.
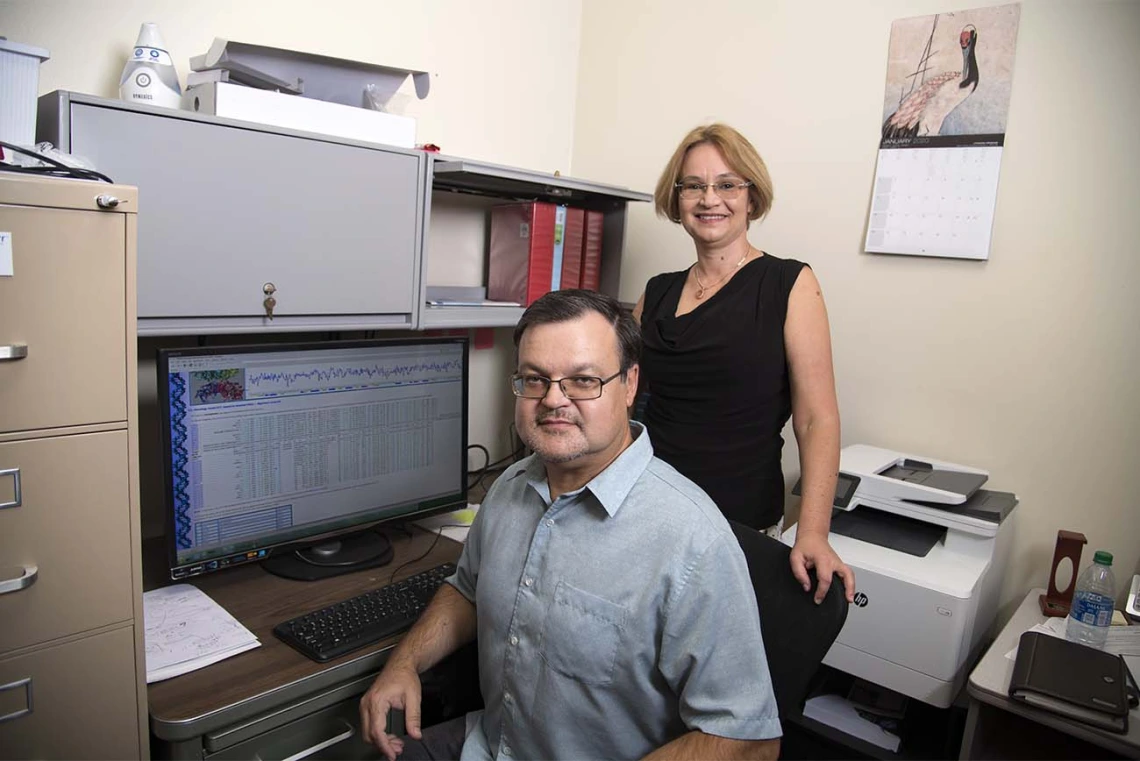
Drs. Ruslan Rafikov and Olga Rafikova study the genetic and metabolomic biomarkers of pulmonary hypertension.
Deadly if not treated, pulmonary hypertension can be easy to miss. Shortness of breath and difficulty exercising are among its symptoms – the same as those indicating a number of other respiratory and cardiovascular conditions.
The standard procedure to detect the disease is a pulmonary artery catheterization, an invasive test where a tube is threaded from a vein in the neck or groin to the heart’s right ventricle and into the pulmonary artery to measure pressure. High blood pressure in that area indicates pulmonary hypertension.
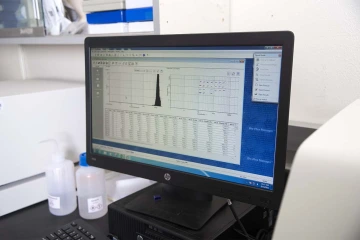
Using technology, artificial intelligence and detective work, researchers at the College of Medicine – Tucson built original algorithms to help doctors more quickly and efficiently diagnose pulmonary hypertension.
Working in the Division of Endocrinology at the University of Arizona College of Medicine – Tucson, the two researchers have created algorithms that can detect the tell-tale metabolites associated with the condition in blood samples, speeding diagnosis, providing a quicker path to treatment and potentially adding years to patients’ lives.
“By creating machine learning techniques or algorithms, we are teaching the computer how to separate metabolite data and determine what’s significant,” Dr. Rafikov said. “Using data, we are allowing for quicker and less invasive ways to assess patients. And that is the goal of our work as we move into the future.”
About 200,000 new cases of pulmonary hypertension are diagnosed every year in the United States, with treatment costs ranging about four to five times higher in patients with the condition than those without, according to a recent report in the American Journal of Managed Care. College of Medicine – Tucson Associate Professor Franz Rischard, DO, estimates the disease occurs at twice the national rate in southern Arizona.
Where machine learning and medicine meet
Drs. Rafikov and Rafikova’s research focuses on the expression of metabolites in cells that are under stress. Metabolites are micro-molecules that drive biochemical activities in cells, such as signaling, stimulatory and inhibitory functions in the body. These small molecules include lipids, proteins and amino and nucleic acids that are building blocks when a cell is in regeneration mode.
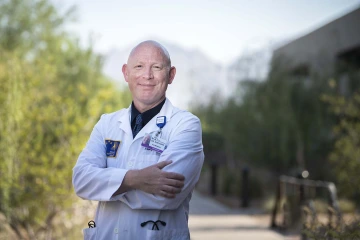
Franz Rischard, DO, says southern Arizona sees twice the national rate of pulmonary hypertension.
Analyzing data from blood samples with a computer algorithm, Drs. Rafikov and Rafikova zeroed in on shifts in about a dozen metabolites that signal early diagnosis.
They then created a machine-learning algorithm – a set of instructions for the computer to scan for metabolites in the blood – to detect the presence of pulmonary hypertension, eventually developing a metabolic profile to hone in on 11 specific metabolites out of tens of thousands in the body.
“It’s similar to how our brains learn to identify a specific image,” Dr. Rafikov explained. “You may need many images before the computer recognizes what you’re teaching it to see.”
The research partners also had to teach the computer to tell the difference between healthy and diseased samples, as well as the differences in samples for other conditions a patient might suffer from, such as diabetes, cancer or other lung and heart diseases.
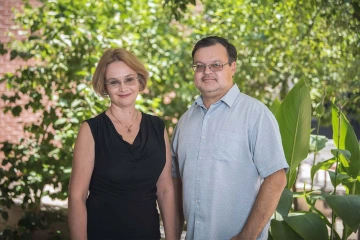
Drs. Rafikova and Rafikov hope to develop additional panels to diagnose other diseases based on their metabolic signatures.
Expanding their work
After further refinements, Drs. Rafikov and Rafikova hope to provide their 11-metabolite panel to physicians to screen patients with mild pulmonary hypertension symptoms and allow earlier diagnosis. They also plan to develop metabolite panels to improve diagnoses for other diseases by identifying different metabolic signatures.
“We want to develop our own unique algorithm for a multi-level, multi-disease focus that would, from the very beginning, approach each patient as if the system understands there might be several conditions in one person,” Dr. Rafikova said.
Contact
David Mogollón
520-275-6931
mogollon@arizona.edu