Integrating AI into research may revolutionize outcomes
Health Sciences researchers encourage its use, but caution that it takes quality data and human supervision to keep “hallucinations” at bay.

Using artificial intelligence in research can help make some tedious tasks easier but ensuring the data is free of biases and misinformation can aid in accuracy.
Photo by Getty Images
Researchers in the field of health sciences are harnessing the power of artificial intelligence to revolutionize their approach to understanding and combating diseases. Across laboratories and institutions worldwide, AI algorithms are now not only assisting but often leading the charge in analyzing vast datasets, predicting patient outcomes and even uncovering novel therapeutic targets. This transformative partnership between human ingenuity and machine learning promises to reshape the landscape of health care, offering unprecedented insights and potential solutions to some of the most challenging medical mysteries of our time.
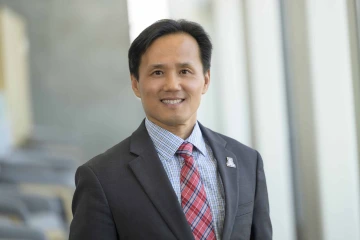
Brian Ahn, PhD, dean of the U of A College of Nursing
Photo by Kris Hanning, U of A Health Sciences Office of Communications
I did not write that paragraph. The artificial intelligence application in ChatGPT did. And while allowing a large language model like ChatGPT to sort through my interviews and synthesize the key points of everyone I interviewed would make my job much easier, is it the ideal or ethical way to present research to an audience?
“AI has the potential to revolutionize teaching, research and problem-solving by enhancing education, advancing research capabilities and improving patient care outcomes,” said Brian Ahn, PhD, dean of the University of Arizona College of Nursing. “Embracing AI responsibly and ethically can lead to significant advancements in nursing practice and health care as a whole.”
Ahn is uniquely positioned to understand this impact. In addition to his extensive nursing research, he has a Bachelor of Engineering degree in electrical engineering from the University of Seoul College of Engineering and a Master of Science degree in Electrical and Computer Engineering from the University of Florida College of Engineering. Ahn currently leads an NIH R01 study that integrates brain AI digital technology into pain and symptom management in older adults.
“The rapid advancement of health care technology, such as machine learning and artificial intelligence, requires the integration of these new technologies into our education and research programs,” Ahn said. “Our college is in the process of establishing a new nursing engineering program and ‘Center for Health and Technology’ to incorporate computer technology into nursing education and research.”
It all starts with good data
AI is a part of computer science that uses computers to do tasks that have historically required a human to complete, like problem-solving or simulating human capabilities, such as analyzing data or translating language. For researchers, sorting large data sets can be onerous work. But utilizing AI can cut that effort drastically.
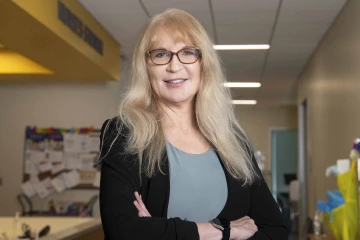
Janine Hinton, PhD, an associate clinical professor and director of the Steele Innovative Learning Center in the College of Nursing
Photo by Kris Hanning, U of A Health Sciences Office of Communications
While images of Skynet might come to mind for some people when the words “AI” and “simulating human capabilities” are combined in one sentence, the ability to sort through large amounts of information, seeking patterns or even holes in the data, can free up researchers to focus on other parts of problems.
“Most of our research has been focused on assessing the current capabilities of AI or large language models,” said Christopher Edwards, PharmD, an associate clinical professor at the R. Ken Coit College of Pharmacy.
Edwards and his colleagues Bernadette Cornelison, PharmD, and Brian Erstad, PharmD, recently worked on a project that examined the accuracy of Chat GPT in providing patient-facing information, particularly on common questions patients should ask their pharmacist when they fill a new prescription. The research assessed the output of the model for accuracy and completeness to see if it was generating quality information. It was looking to see if the AI was “hallucinating,” which is when an AI provides inaccurate or misleading information. The AI may have incomplete or incorrect data; there may be biases in the data or the old “garbage in, garbage out” with false information in the data set.
“It can be very helpful writing learning objectives, test questions and editing,” said Janine Hinton, PhD, an associate clinical professor and director of the Steele Innovative Learning Center in the College of Nursing. “But you have to be very careful. It’ll give you a reference that doesn’t make sense. It’s just hallucinating.
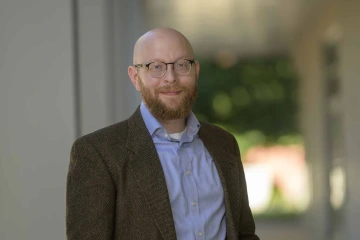
Christopher Edwards, PharmD, an associate clinical professor at the R. Ken Coit College of Pharmacy
Photo by Kris Hanning, U of A Health Sciences Office of Communications
“But it will also present ideas that maybe I hadn’t originally thought of and just help me get my work done faster. I know that there are people who really want to get it moving in health care, but there are challenges with confidentiality, with clinical decision-making. But I do think there are a lot of ways to blend it with other tools and our expertise to get our work done.”
Hinton, who is also a member of the BIO5 Institute, explained that the College of Nursing uses AI to model a patient in simulation training at the Gilbert campus. The AI monitors and provides cues and clues to students to help them recognize interventions faster.
AI is much like gold mining
Travis Wheeler, PhD, an associate professor at the Coit College of Pharmacy whose doctoral degree is in computer science from the U of A, compared AI to mining.
“The power of AI comes from combining lots of training data with advanced techniques for learning to extract patterns from the data,” he said. “It’s a bit like mining. You might have a process that sifts through the dirt to extract big pieces of gold or minerals, but if there’s nothing valuable in the dirt, you won’t get anything out of it. That’s like feeding bad data to an AI model, and is what we mean by ‘garbage in.’ But if the dirt has a ton of really useful stuff and your method only extracts the gold, there are still some useful rare earth materials that you tossed away because you were using bad technology. You would be missing other things that are in there.

Photo by Getty Images
“With better methods, you can extract more material out of that dirt. As AI methods advance, it’s like building better mining methods, where you can extract more information out of the data you are given. It’s not only the quality of the data but also the tools used to extract the information out of that data.”
Wheeler is a lynchpin at the Health Sciences for AI work. He designs AI architectures able to ingest data while accounting for biases or missing data, to allow the artificial intelligence models to learn to tell the difference between that hypothetical gold-painted rock and a real gold nugget. He has spent more than 25 years in research in designing algorithms, statistical models, and software for problems motivated by biological data.
“The key idea behind AI and machine learning is that these models learn to perform classification or prediction tasks based on patterns extracted from the training data,” he said. “The challenge of the whole thing is both developing these large data sets that will provide the necessary information and then developing the kind of neat computational architectures that are capable of learning from those data.”
Beyond Chat GPT and crunching numbers
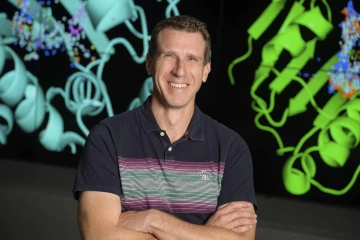
Travis Wheeler, PhD, an associate professor at the R. Ken Coit College of Pharmacy
Photo by Kris Hanning, U of A Health Sciences Office of Communications
But much like mining, recognizing when you have a gold nugget versus a gold-painted rock is important for researchers using AI.
“You’re taking data that is not completely vetted, not completely curated, so we have to learn how to mitigate bad-quality data,” said Nirav Merchant, director of the Data Science Institute and a member of the university’s AI Access & Integrity Working Group.
Any chef can tell you that your dinner is only as good as the ingredients you use. It’s the same with using AI in research. But AI can move beyond crunching large data sets.
Allan Hamilton, MD, the executive director of the Arizona Simulation Technology and Education Center, is researching how AI can be used as a coaching tool.
“AI should move the educational experience up almost to real time. It’s coaching each individual exactly how they need to be coached,” he said.
One way Hamilton uses AI is through a bot that can respond in real time, using a variety of emotions, to help coach new physicians. The second part of his research is finding ways AI can free up physicians.
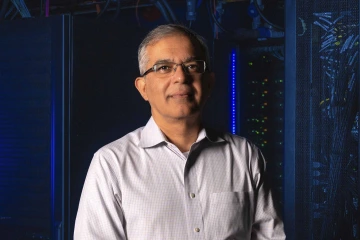
Nirav Merchant, director of the Data Science Institute
Photo by Noelle Haro-Gomez, U of A Health Sciences Office of Communications
“Thirty percent of a doctor’s time is paperwork,” Hamilton said. “We know AI can do a lot of paperwork for us. How do we reapply that time? Hopefully the answer would be, You put it to good human use!”
For instance, he described a scenario in an intensive care unit where AI is monitoring a patient.
“AI could make predictions about which patients were more likely to need rapid response, but it might also say the whole ICU team doesn’t need to respond; you can send just two people,” Hamilton said. “It ended up being 245% better at identifying who was likely to need rapid response than the hospital teams determined.
“It’s like, is it safer for me to be on the road with GPS and not looking around trying to figure out where I am going? Yeah, it is.”
Hamilton, though, cautions against health-care providers relying too much on AI.
“I always say to students, ‘Bots don’t go to jail. Doctors do.’ So, if a patient dies because a physician did precisely what a bot told them to do rather than what their training guided them toward, the human will be held responsible,” he said.
A good tool when used properly
The researchers agreed that being transparent when AI or chatbots have been used in research is necessary for research integrity. As useful as it is to have AI write a draft of an abstract or study results, researchers need to be up front that it was utilized.
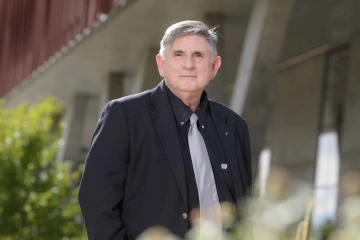
Allan Hamilton, MD, the executive director of the Arizona Simulation Technology and Education Center
Photo by Kris Hanning, U of A Health Sciences Office of Communications
Justin Starren, MD, PhD, the director of the Center for Biomedical Informatics and Biostatistics at the University of Arizona Health Sciences, compared relying on AI to his time in New York, where he lived on a wooded lot.
“That meant I had a chain saw,” he said. “A chain saw is a great tool, but if you don’t really understand how it works, you’re probably going to get the nickname ‘Stubby.’ The current AI tools are like chain saws – they can cut through a huge amount of data really fast. And they can figuratively take an arm off equally as fast. They can be profoundly powerful, but profoundly stupid. The risk is that we know very well that people tend to believe computers, even when they are wrong. It’s an extremely powerful tool.”
The issues of ensuring that good-quality data is being used, what the AI has been trained on and what tweaks were put into the system after it was trained are critical to minimizing racial bias or made-up answers.
“These tools can be great proofreaders, great hypothesis generators and do universe matrix analysis to find the hole in the data, but we need to be transparent about their use,” Starren added.
“I prefer to look at it as augmented intelligence rather than artificial intelligence,” said Merchant. “If you know how to use it, you’re going to be productive with it, but if you don’t know how to engage with it, you’re going to always try and find a nail for that hammer. So, stop thinking of AI as the hammer and just look where you can use it. Where can you use the automation that comes with some AI components?”
“The power of AI comes from combining lots of training data with advanced techniques for learning to extract patterns from the data.”
Travis Wheeler, PhD, an associate professor at the R. Ken Coit College of Pharmacy
So much more to come with AI
Merchant cautioned researchers that chat tools and large language models are only the tip of the iceberg when it comes to using AI in research.
“People are building really purposeful analysis methods and tools that are constantly coming out,” he said. “Pay attention to those and see how we can use them because they will readily improve your science.”
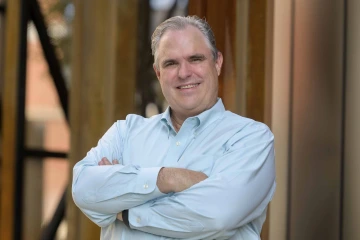
Justin Starren, MD, PhD, the director of the Center for Biomedical Informatics and Biostatistics at the University of Arizona Health Sciences
Photo by Kris Hanning, U of A Health Sciences Office of Communications
For most of the Health Sciences researchers, however, AI, or more specifically, Chat GPT, can’t replace the human touch when “swimming in the sea of language,” as Starren put it. Often, AI-written content just doesn’t read “human.”
“I tried to use it to help write my wedding vows,” Edwards said. “And then I read it, and thought, ‘This is mechanical garbage.’ I don’t want to sound like this, because this is terrible. It gave me a starting point, though, and spurred my creative juices.”
Much like the start of this story, I asked ChatGPT to wrap it up as if it were writing it:
As AI continues to evolve and integrate into health sciences, its role as both a tool and a collaborator will only expand. The potential benefits are immense, from streamlining data analysis to enhancing patient care and education. However, the conversation around its ethical use and integration is equally crucial. As researchers and practitioners navigate this new frontier, they must balance the efficiency AI offers with the nuanced human touch that remains indispensable in healthcare. The ongoing challenge will be to harness AI’s capabilities responsibly, ensuring that it complements rather than compromises the human elements of empathy, creativity and critical thinking.
Learn more
To learn more about the University of Arizona’s artificial intelligence resources and tools, a website outlining standards, usage and AI-related courses is available. The AI working group holds regular meetings on AI-related topics.