Data Mining Collaboration Brings Researchers Together
Researchers at UArizona Health Sciences and the Eller College of Management are using data mining to improve medical communication and diagnoses.
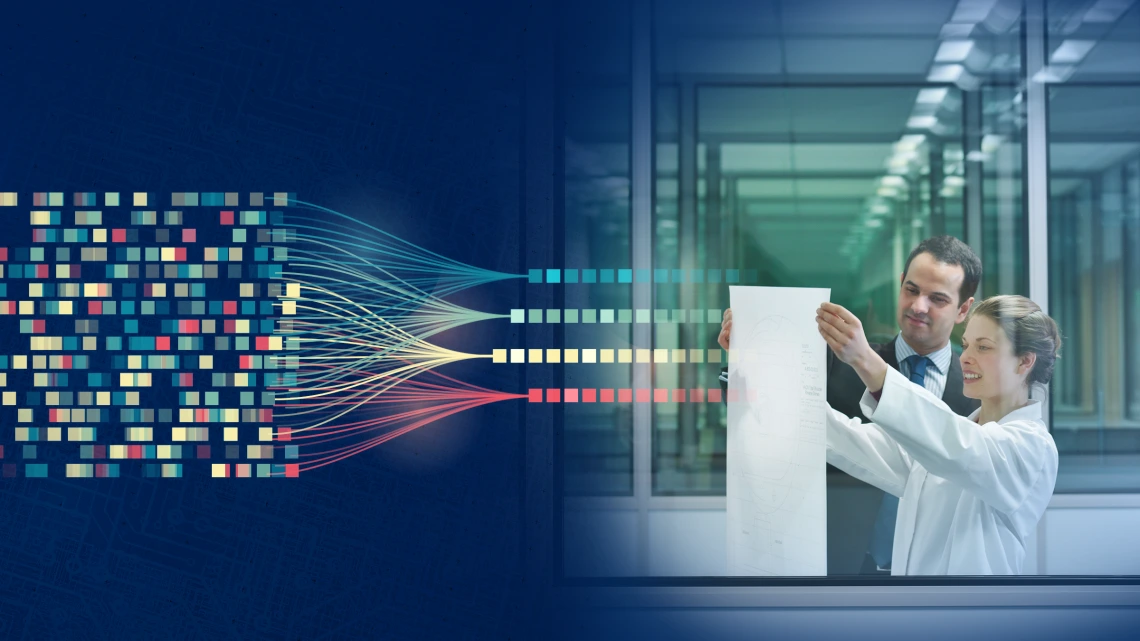
If many hands make light work, a collaboration of like minds can make that work even lighter.
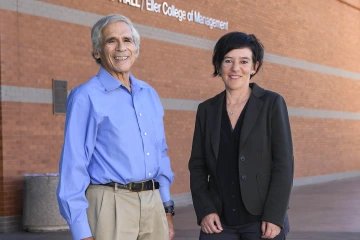
(From left) Phil Harber, MD, MPH, a professor in the Mel and Enid Zuckerman College of Public Health, and Gondy Leroy, PhD, a professor in the Eller College of Management, are collaborating on a study to improve text-to-speech machine learning for more effective communication between clinicians and patients.
Dr. Leroy is working with Sydney Rice, MD, a professor and chief of the Division of Genetics and Developmental Pediatrics at the UArizona College of Medicine – Tucson; Jennifer Andrews, PhD, an associate professor of pediatrics and director of research for both the Division of Genetics and Developmental Pediatrics and the Division of Pediatric Cardiology Congenital Heart Program; Maureen Kelly-Galindo, MS, RN, a pediatric genetics nurse at Banner – University Medical Center Tucson and research data manager for the Division of Genetics and Developmental Pediatrics; and Win Burleson, PhD, a professor in the UArizona College of Social and Behavioral Sciences’ School of Information who focuses on human-computer interaction. Their goal is to more quickly identify children with autism spectrum disorder (ASD) from cues left in clinician notes included in EHRs.
The second research team includes Philip Harber, MD, MPH, a Mel and Enid Zuckerman College of Public Health professor, Jeff Stone, PhD, a Distinguished Professor of Psychology in the UArizona College of Science, Steve Rains, PhD, a professor of communication in the UArizona College of Social and Behavioral Sciences, and David Kauchuck, PhD, an associate professor and computer science chair at Pomona College. They’re investigating implications of new audio communication technologies for understanding health messaging and allowing patients to better manage their medical conditions.
Both federally funded studies hinge on natural language processing, a subfield of linguistics, computer science and artificial intelligence based on interactions between computers and human language. The studies focus on analyzing large amounts of data using machine learning with algorithms developed to target specific words or phrases.
Finding ways to speak the same language
Initially, though, the collaborative efforts had to surmount team members’ own language challenges.
“We were so different. At first, I think, we had to learn each other’s language,” Dr. Leroy said of working with Dr. Rice’s autism team. “For example, what I mean by precision means something else in Dr. Rice’s world, and there’s sensitivity around all these things related to autism. We had some data, and we knew where we spoke a different language or had different priorities. And I think it’s kind of nice that we are now a team.”
Dr. Rice agreed. “We come from such different backgrounds that it’s always interesting. I learn something every time we talk because we live in such different worlds,” she added.
“We come from such different backgrounds that it’s always interesting. I learn something every time we talk because we live in such different worlds.”
Sydney Rice, MD
Dr. Rice underscored that ASD diagnoses have increased as understanding of autism has grown and other conditions were rolled into “the spectrum” of ASD.
“Today, though, we’re still acting, as diagnosticians and clinicians, like autism is diagnosed in 1 in 10,000 children, when now it’s 1 in 44 8-year-olds that are identified with ASD. Our clinical workforce can’t manage this. We need data mining to move us forward.
“It used to be when I started my career, autism was a rare condition. But it is now common and we need to develop better ways to work because children are waiting too long to get a diagnosis,” Dr. Rice said.
By using Dr. Leroy’s machine-learning methods, the team’s objective is to simplify initial assessments to identify who needs additional, more personal evaluation for ASD.
“We're trying to figure out more effective ways of obtaining and cleaning that data and using humans as a double-check or a quality control rather than an initial pass,” Dr. Andrews said.
She and Kelly-Galindo, who served as a U.S. Centers for Disease Control and Prevention clinician reviewer in the Autism and Developmental Disabilities Monitoring (ADDM) Network, provide high-quality records to train Dr. Leroy’s algorithm programs how to label ASD cases more accurately.
Text simplification for audio comprehension
Dr. Harber’s text-audio research team seeks to improve patient health by increasing adherence to physicians’ treatment plans.
“You go in, a nurse gathers some data, a doctor sees you and then some information needs to be shared with you,” Dr. Harber said. “That may be giving you a summary of your visit record, but it also may be giving you a document about this is how you should take your pill, use your inhaler or watch your diet. It also can provide you a referral for follow-up care. But it goes beyond just an after-visit summary.”
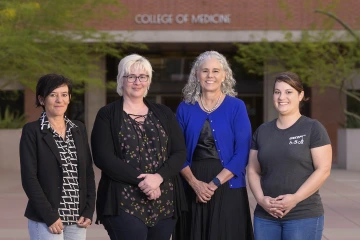
(From left) Gondy Leroy, PhD, Jennifer Andrews, PhD, Sydney Rice, MD, and Maureen Kelly-Galindo, MS, RN, of the College of Medicine – Tucson’s Division of Genetics and Developmental Pediatrics, are working together to more quickly diagnose autism based on language used in clinician notes on patient evaluations.
The crux, Dr. Harber noted, is people are different. Age, education level, language, cultural or ethnic influences, and preferred style of communication all impact how medical advice should be shared in the best manner.
“We’re developing a system to take medical text and put it in an audio form and optimize it – not just push it through a text-to-speech converter, but put it into an optimal form that can be effectively used by the individual. And we want to be able to do this in a way that is not effort-intensive for the provider,” he said.
In that way, patients are more likely to understand and follow clinician instructions.
The audio aspect, Dr. Leroy said, is one she’s excited about if only because the explosion in podcasts in recent years has shown people do retain information from audio formats as well as text.
“People more and more listen to information on their phones and from smart speakers, car speakers, earbuds and all that. And we’re, as far as I know, still the only ones focusing on audio and simplifying information in audio for people to understand that better,” Dr. Leroy added.
Building blocks for future breakthroughs
Any assistance in identifying children who need help is welcome, Dr. Rice said. As lead clinician and principal investigator also of the UArizona Children’s Postinfectious Autoimmune Encephalopathy Center of Excellence, she sees a variety of patients with complicated mental and behavioral issues like ASD with no easy test or diagnostic codes to pinpoint their condition. Thus, she acknowledged, an automated system to cull through EHR verbiage and alert doctors to possible diagnoses would be extremely helpful beyond just the need to more quickly identify autism.
Dr. Leroy said unique aspects of both studies show that data mining, machine learning and natural language processing offer ways to improve efficiencies in how medicine is practiced.
“This is a great opportunity to do bigger things. It allows us to set ourselves up to do better for different types of communities and for science and for research,” Dr. Leroy said. “We are going to accomplish a lot.”